【佳学基因检测】EAMA:基因组实验中大规模同时假设检验的经验调整荟萃分析
品牌肿瘤基因检测688套餐解剖
与专家交流肿瘤基因检测全面性的标准与实施方案《肿瘤突变基因检测与个性化治疗方案的制定》《PLoS One》在. 2017 Oct 31;12(10):e0187287.发表了一篇题目为《EAMA:基因组实验中大规模同时假设检验的经验调整荟萃分析》肿瘤靶向药物治疗基因检测临床研究文章。该研究由Sinjini Sikdar , Somnath Datta , Susmita Datta 等完成。促进了肿瘤的精准治疗与个性化用药的发展,进一步强调了基因信息检测与分析的重要性。
如何提高肿瘤基因检测机构的排名临床研究内容关键词:
高通量,基因组,数据集,统计分析,大规模
肿瘤靶向治疗基因检测临床应用结果
高通量基因组分析的最新发展开辟了同时测试成百上千个基因的可能性。然而,在这种大规模的多重测试框架中坚持关于测试统计的零分布的常规统计假设有可能导致不正确的显着性测试结果和有偏见的推理。当一个人将不同独立基因组实验的结果与可能最终发现重要基因的严重错误发现相结合时,这个问题会变得更糟。在本文中,我们开发了一种元分析方法,通过对单个测试统计数据和 p 值的经验调整,结合来自涉及大规模多重测试框架的不同独立实验的 p 值。尽管它基于各种现有的想法,但这种特定的组合是新颖的并且可能有用。通过模拟研究和真实的基因组数据集,我们表明我们的方法在准确识别真正重要的基因组方面优于标准的显着性检验荟萃分析方法。
肿瘤发生与复发转移国际数据库描述:
Recent developments in high throughput genomic assays have opened up the possibility of testing hundreds and thousands of genes simultaneously. However, adhering to the regular statistical assumptions regarding the null distributions of test statistics in such large-scale multiple testing frameworks has the potential of leading to incorrect significance testing results and biased inference. This problem gets worse when one combines results from different independent genomic experiments with a possibility of ending up with gross false discoveries of significant genes. In this article, we develop a meta-analysis method of combining p-values from different independent experiments involving large-scale multiple testing frameworks, through empirical adjustments of the individual test statistics and p-values. Even though, it is based on various existing ideas, this specific combination is novel and potentially useful. Through simulation studies and real genomic datasets we show that our method outperforms the standard meta-analysis approach of significance testing in terms of accurately identifying the truly significant set of genes.
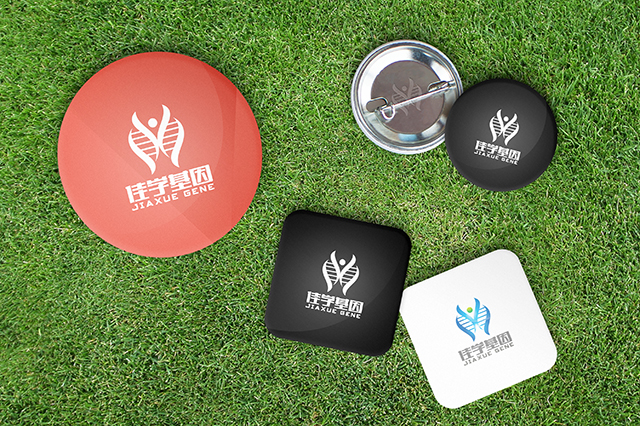
(责任编辑:佳学基因)