【佳学基因检测】微阵列基因检测差异表达基因的鉴定和错误发现率
千万不要做基因检测合理吗
挖掘肿瘤基因组学个性化药物选择时,做了一个记录,《Curr Opin Lipidol》在. 2007 Apr;18(2):187-93.发表了一篇题目为《微阵列研究中差异表达基因的鉴定和错误发现率》基因检测先进技术及各种不同技术的比较优势临床研究文章。该研究由Arief Gusnanto, Stefano Calza, Yudi Pawitan等完成。促进了微阵列芯片基因检测技术的传播和比较,进一步强调了不同基因检测技术的选择及合理应用。
基因检测技术的临床研究内容关键词:
微阵列,基因芯片,基因检测,技术优势
微阵列及高密度芯片基因检测临床应用结果
审查基因解码基因检测的研究目的:突出微阵列数据分析在识别差异表达基因方面的发展,特别是通过控制错误发现率。最近的发现:高通量技术(如微阵列)的出现引发了两个基本的统计问题:多重性和敏感性.基因解码基因检测专注于识别差异表达基因的生物学问题。首先,由于测试了数以万计的假设而产生了多重性,使标准 P 值变得毫无意义。其次,已知的最佳单次测试程序(例如 t 测试)在高度多重测试的情况下表现不佳。处理多重性的标准基因解码基因检测的研究方法在微阵列环境中过于保守。错误发现率概念正迅速成为取代 P 值的关键统计评估工具。基因解码基因检测回顾了错误发现率基因解码基因检测的研究方法,并认为它对微阵列数据更明智。基因解码基因检测还讨论了一些基因解码基因检测的研究方法来考虑来自微阵列的额外信息以提高错误发现率。总结:关于如何使用错误发现率框架代替经典 P 值来分析微阵列数据的共识越来越多。需要进一步研究原始数据的预处理,例如标准化步骤和过滤,以及寻找最敏感的测试程序。
微阵列及高密度芯片基因检测国际数据库描述:
Purpose of review: To highlight the development in microarray data analysis for the identification of differentially expressed genes, particularly via control of false discovery rate.Recent findings: The emergence of high-throughput technology such as microarrays raises two fundamental statistical issues: multiplicity and sensitivity. We focus on the biological problem of identifying differentially expressed genes. First, multiplicity arises due to testing tens of thousands of hypotheses, rendering the standard P value meaningless. Second, known optimal single-test procedures such as the t-test perform poorly in the context of highly multiple tests. The standard approach of dealing with multiplicity is too conservative in the microarray context. The false discovery rate concept is fast becoming the key statistical assessment tool replacing the P value. We review the false discovery rate approach and argue that it is more sensible for microarray data. We also discuss some methods to take into account additional information from the microarrays to improve the false discovery rate.Summary: There is growing consensus on how to analyse microarray data using the false discovery rate framework in place of the classical P value. Further research is needed on the preprocessing of the raw data, such as the normalization step and filtering, and on finding the most sensitive test procedure.
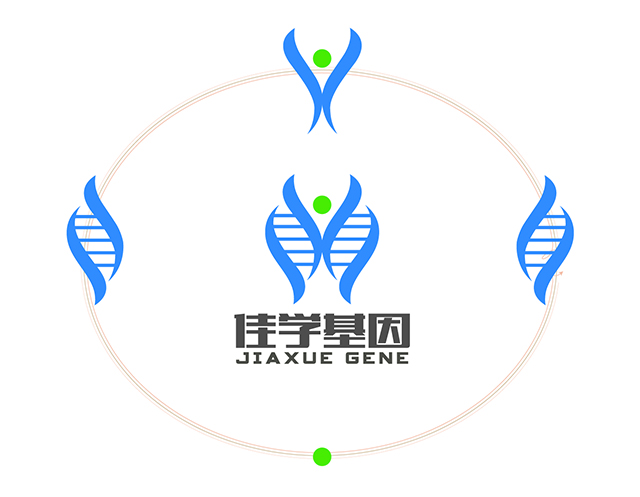
(责任编辑:佳学基因)